
Manufacturing Analytics: Understanding Machine
New types of sensors and other connected devices are fueling this revolution by generating enormous amounts of data that offers new opportunities to monitor and control production equipment and supply chains. That leads to greater efficiency: manufacturers are using these insights to improve processes, create big jumps in productivity, and reduce time-to-market by as much as 50%.
Unfortunately, the under-powered business intelligence (BI) tools that have entered the marketplace in the last decade are not going to get your manufacturing company where it needs to go. Yes, their beautiful dashboards can seem compelling as they give everyone from production engineers to product managers a snapshot answer for “What is happening?” on the factory floor and elsewhere.
But that isn’t enough if you plan to be a next-gen manufacturer. The real question you need to be answered by your manufacturing analytics tool is “Why is it happening?”
METHODS
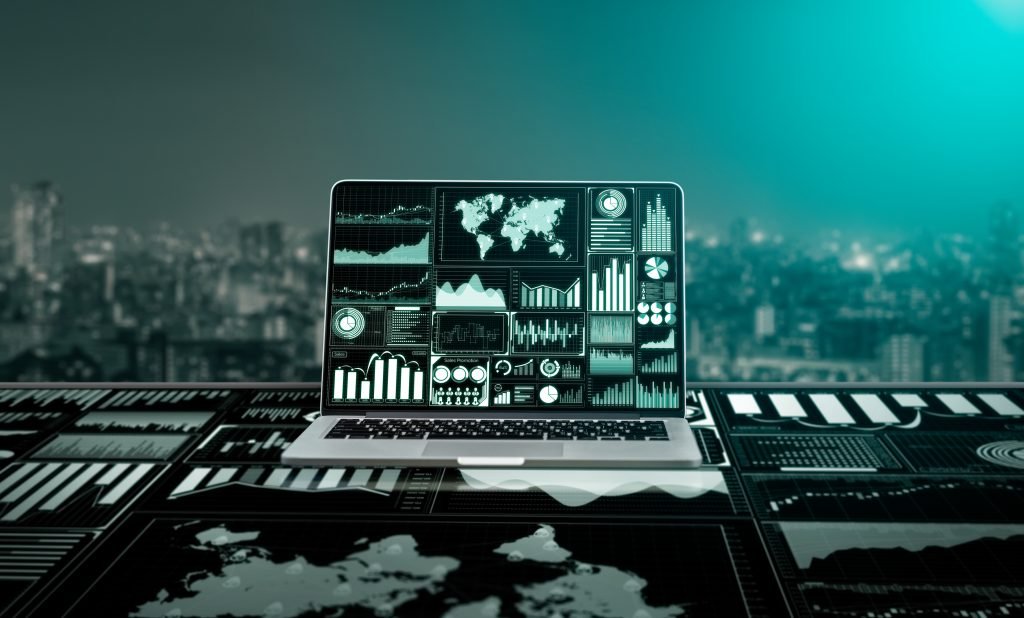
In a typical Data Analytics project the workflow can be summarized by the CRISP-DM process:
Business Understanding: Ask first the questions, then look for the data (not the other way around). You need to have a deep understanding of business. Many consulting companies fail to understand complex customer business. Lantek knows the way!
Data Preparation: Data quality is critical. Most of the time invested (around 60% to 80%) in a Data Analytics project deals with data collection, preparation, profiling, cleaning, and wrangling to prepare a table (dataset) used later with Machine Learning. If the data quality is low, then you get — garbage in and garbage out!
Modeling: Machine learning (ML) is the motor that drives data science. Each ML method takes in data, turns it into a Data Model, and spits out an answer. ML methods do the part of data science that is the trickiest to explain. That is where the mathematical magic happens.
Data Models assume that the future will behave in a similar way as the past. Unforeseen events, like a world financial crisis or a natural disaster, cannot be integrated into the data model.
It is worth mentioning that the data models always make a prediction with a probability associated with it. We are all used to weather forecasting and there the associated probability is always present.
Evaluation: At this stage, you’ll assess the value of your models for meeting the business goals and consider the risks when the model probability is low or it classifies with False-Positives and False-Negatives.
Deployment: When your model is ready to use, you will need a strategy for putting it to work in your business. An often-overseen issue is that this deployment is only possible with a high company maturity and workers willing to adopt the new data-driven processes.
APPLICATIONS

Data Analytics Types and their use in Manufacturing Analytics:
Descriptive: What is happening (Visualization)
With descriptive analytics, also well known as Business Intelligence, the historical data is visualized with powerful and interactive software tools. Decision-makers can identify radically more about their businesses and translate that knowledge into improved decision making. Dashboards such as the OEE are created in real-time as are found in Manufacturing Analytics solutions.
Diagnostic: Why it is happening (Root cause analysis, inference)
Identifies the cause(s) of a manufacturing problem, such as why a process has a bottleneck or why the quality is so low.
Predictive: What is likely to happen (Forecasting)
With predictive analytics, there is a look into the future. Predicted Machine Maintenance, Inventory prediction, Demand forecasting are typical applications.
Prescriptive: What do I need to do (Automation)
Automated manufacturing systems need to make real-time decisions based on data and take actions, fully automated, creating an autonomous cyber-physical system.
CONCLUSION

Driving productivity by enhancing productivity has been the crux of every industrial revolution. The fourth industrial revolution brought about waves of transformations that resulted in an increase in productivity in both production and management systems.
From a business viewpoint, the goal of every manufacturing company is to be able to manufacture goods at lower costs. To achieve this goal and improve collaboration between supply chain, manufacturing, and sales operations, it’s crucial to rethink the production processes and bring more automation to factories. The manufacturing analytics solutions will help companies to pioneer increasingly efficient ways to produce and transport goods while thinking beyond productivity and cost savings. Through our industrial analytics solutions we also aim to help companies:
- Capitalize on data as an asset
- Deliver quality products
- Fine-tune production operations